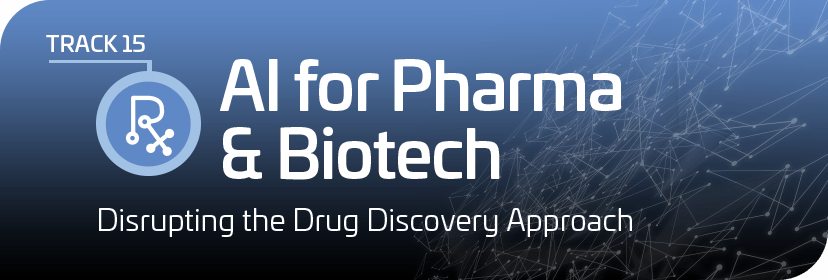
One of the biggest bottlenecks in drug development is in the early research stage. This stage is time needed to go from identifying a potential disease target to testing a drug candidate’s probability of hitting that target. This stage can take
four to six years. Ambitious AI techniques are aiming to compress this process to one year. As of August 2018, over 25 pharmaceutical companies and over 95 startups are using artificial intelligence for drug discovery. Time to develop new life-saving
drugs can be drastically reduced by using AI. The Inaugural AI for Pharma and Biotech track will discuss opportunities that biopharma organizations are using to harness the power of AI and machine learning technologies to maximize and accelerate drug
discovery efforts from early stage to adoption to practical application. Presentations will also discuss challenges of these technologies being sophisticated enough to make sense of complex medical data.
Final Agenda
Tuesday, April 16
7:00 am Workshop Registration Open and Morning Coffee
8:00 – 11:30 Recommended Morning Pre-Conference Workshops*
W4. AI for Pharma
12:30 – 4:00 pm Recommended Afternoon Pre-Conference Workshops*
W11. Digital Data Strategy for the Lab
* Separate registration required.
2:00 – 6:30 Main Conference Registration Open
4:00 PLENARY KEYNOTE SESSION
Amphitheater
5:00 – 7:00 Welcome Reception in the Exhibit Hall with Poster Viewing
Wednesday, April 17
7:30 am Registration Open and Morning Coffee
8:00 PLENARY KEYNOTE SESSION
Amphitheater
9:45 Coffee Break in the Exhibit Hall with Poster Viewing
10:50 Chairperson’s Remarks
Richard Harrison, PhD, Products – Life Sciences, Senior Manager, Accenture
11:00 Next Generation Cognitive Supercomputing and Its Impact on Precision Medicine: Challenges, Trends and Opportunities
Edmon Begoli, PhD, Chief Data Architect, Oak Ridge National Laboratory
The impact of AI methods and big data technologies in pharma research and genomic medicine to advance precision medicine initiatives are taking on more importance. AI is becoming key player in the convergence of medical data and computer technologies.
This talk gives a big picture viewpoint on the evolvement of AI’s role, what the key drivers and challenges are, and trends to look for during the next 10-15 years.
11:30 From Hype to Reality: Data Science Enabling Personalized Medicine
Holger Fröhlich, PhD, Director and Head of Data Science Enablement, R&D Informatics, UCB BioSciences GmbH
Personalized, precision, P4, or stratified medicine is understood as a medical approach in which patients are stratified based on their disease subtype, risk, prognosis, or treatment response using specialized diagnostic tests. The key idea is to base
medical decisions on individual patient characteristics, including molecular and behavioral biomarkers, rather than on population averages. Personalized medicine is deeply connected to and dependent on data science, specifically machine learning (often
named Artificial Intelligence in the mainstream media). While during recent years there has been a lot of enthusiasm about the potential of ‘big data’ and machine learning-based solutions, there exist only few examples that impact current
clinical practice. The lack of impact on clinical practice can largely be attributed to insufficient performance of predictive models, difficulties to interpret complex model predictions, and lack of validation via prospective clinical trials that
demonstrate a clear benefit compared to the standard of care. In my talk, I will review the potential of state-of-the-art data science approaches for personalized medicine, discuss open challenges, and highlight directions that may help to overcome
them in the future.
12:00 pm Harness AI and Redefine Precision Medicine Infrastructure with Dell EMC
Sanjay Joshi, Industry CTO, Healthcare Dell EMC, Global CTO Office, Dell
EMC
AI is making data your top asset; but unlocking its value isn’t a one-click action. Without AI, it’s difficult to generate value from data of lifestyle information from IoT devices, TBs of genomics data, and population studies. We’ll
focus on key attributes of AI solutions that drive success in Life Sciences: enable faster diagnoses, affordable genomics, and improved patient outcomes.
12:30 Session Break
12:40 Luncheon Presentation I: Productionising AI – Moving Beyond “Fire and Forget” to Predictable, Predictive Algorithms
Tim Miller, Vice President, Life Sciences Platform Solutions, Elsevier
Everybody is “doing AI” in Life Sciences right now, but how do we know we are doing it right? Are we picking the best models for our problem? Are we getting the quality of data we need? And, are we able to translate successful AI experiments
into productionised capabilities that integrate with our business goals?
1:10 NEW: Luncheon Presentation II: Enabling Perpetual Digital Transformation in Research & Development
Vikram Karakoti, Head, Life Sciences Business Unit, North America, Tata Consultancy Services
Life Sciences companies today are inverting the pyramid by embedding enabling technologies in business platforms. “Enabling Perpetual Digital Transformation in R&D” explores how these digital platforms are re-constructed, and coupled with
an “enterprise agile” R&D organization to yield exponential improvements in speed, capacity, cost, quality and improved outcomes in product research and clinical development; with real-life examples of just how the new paradigm is
being embraced by leading Life Sciences companies.
1:40 Session Break
1:50 Chairperson’s Remarks
Edwin Addison, PhD, MBA, JD, CEO, Cloud Pharmaceuticals
1:55 Analysis of Off-Label Uses of Drugs for Rare Diseases
Matthew Clark, PhD, Director, Scientific Services, Life Sciences, Elsevier
Many rare diseases do not have approved treatments. It may be that drugs approved for other indications may be useful to treat these diseases, but it is difficult to gather information outside of formal publications. However, the FDA adverse event database,
FAERS, captures prescribing indications for all drugs administered to a patient when any adverse event is reported. By mining this data one can see what individual physicians are using to treat diseases and compare to treatments that appear in formal
trials. We will also compare the “real life” treatments to those that can be found using informatics.
2:25 Using AI to Identify and Navigate Relationships and Identify Context for Pharma Data
Peter Henstock, PhD, Machine Learning & AI Technical Lead, Business Technology, Pfizer, Inc.
As our pharma data sets increase in size, the ability to fully utilize them has become more challenging. A baseline approach is search capability provided in various forms from the databases-level queries through enterprise-level results. The limitations
are being able to create the right queries to find all the relevant information without having to craft the perfect queries or sift through 1000s of entries. The approach draws upon text mining, information retrieval and network analysis all behind
a common user interface and is currently being developed for multiple databases.
2:55 Accelerating Drug Discovery
with Generative Design and Active Learning
Ton Van Daelen, Portfolio
Lead, Scientific Informatics, Dassault Systemes
Enabling pharmaceutical and biotech businesses to produce safe, efficacious medicines is key to improving productivity and maintaining competitiveness. By integrating machine-learning approaches with generative molecular enumeration algorithms,
we can transform the traditional Design, Make, Test, Analyze innovation cycle in drug research. LS organizations can achieve business transformation in molecular discovery by improving quality of lead molecules and shortening discovery timelines.
3:25 Refreshment Break in the Exhibit Hall with Poster Viewing, Meet the Experts: Bio-IT World Editorial Team, and Book Signing with Joseph Kvedar, MD, Author, The Internet of Healthy Things℠ (Book will be available
for purchase onsite)
4:00 PANEL DISCUSSION: The Difference between Biomarkers and Diagnostics: It’s Bigger Than You Think
Moderator:
Michael N. Liebman, PhD, IPQ Analytics, LLC and Strategic Medicine, Inc.
Panelists:
Michael Montgomery, MD, Global Executive Director, Incyte Corporation
Jonathan Morris, MD, Vice President, Provider Solutions; Chief Medical Informatics Officer, Real World Insights, IQVIA
Jun Zhu, PhD, Professor, Genetics and Genomic Sciences, Icahn School of Medicine at Mount Sinai
Biomarkers are used to evaluate cancer risk, detect end stage cancer and suggest optimal therapy for specific patients. Recent advances in -omics research continue to enable researchers to classify molecular fingerprints of specific cancers. Discovery
and development of new cancer markers remains a major research focus to improve screening, diagnosis, and treatment but is hampered by the limited knowledge of the details of disease progression. This challenges the ability to identify markers that
may be causal rather than correlative and impacts their use as true diagnostics. By example, literature analysis of 250,000 papers listing biomarkers in cancer yield less than 100 FDA approved diagnostics. Every experiment yields a biomarker; however,
every experiment does not yield a diagnostic that can more accurately drive clinical action. How can we close this gap? It is critical to develop a better understanding of the disease process and how observations from genomics, proteomics, and metabolomics
may impact that process in different ways. This interactive panel will explore experimental and analytic methods, issues and challenges impacting identification, validation, development and implementation in cancer, diagnosis and treatment.
5:30 Best of Show Awards Reception in the Exhibit Hall with Poster Viewing
Thursday, April 18
7:30 am Registration Open and Morning Coffee
8:00 PLENARY KEYNOTE SESSION & AWARDS PROGRAM
Amphitheater
9:45 Coffee Break in the Exhibit Hall and Poster Competition Winners Announced
10:30 Chairperson’s Remarks
Daniel H. Robertson, PhD, Vice President, Digital Technology; Director, Center for Applied Data Sciences, Indiana Biosciences Research Institute
10:40 Making Real World Data Prepared to Apply Machine Learning and AI for Discovery Research
Daniel H. Robertson, PhD, Vice President, Digital Technology; Director, Center for Applied Data Sciences, Indiana Biosciences Research Institute
Although much of the major healthcare systems transition to electronic medical record (EHR) systems to digitally capture the patient data is complete, this data is not readily available or in the form to enable advanced analyses within discovery. Working
with EHR data extracted from a large health information exchange, we have developed a robust and standardized data-cleaning pipeline to produce a clean, high-quality and normalized dataset ready for research. This process is transferable to other
EHR datasets and leverages modern cloud-based big data tools. Finally, this data has been used to enable discovery research including understanding of disease progression, medication pathways, patient stratification, and digital diagnostics.
11:10 Boosting Drug Discovery with Machine Learning
Rishi Gupta, PhD, Senior Research Scientist, AbbVie, Inc.
Abhik Seal, PhD, Senior Data Scientist, AbbVie, Inc.
11:40 NEW: Applying Structure to the Unstructured: Using AI to Distill Insights from Disparate Unstructured Data Sources
Eric Baldwin, PhD, Solution Executive, IBM Watson Health, IBM
Unstructured data is a significant source of cross-disciplinary insight. However, it’s a significant hurdle to manually read, digest and extrapolate a holistic view. Learn how AI applies a common framework to the disparate data using NLP and predictive
analytics to construct a network of known and inferred connections between biological concepts enabling researchers to make informed, cross-silo decisions.
12:10 pm Session Break
12:20 Luncheon Presentation: From Hype to Reality: AI is
a Key Enabler in Accelerating Drug Discovery and Development
Kailash Swarna, PhD, MBA, Industry Principal Director, Global Life Sciences, Accenture
The recent acceleration of interest in the applications of AI and ML in drug discovery and development has created significant perturbation in life sciences R&D. Separating fact from fiction, and demonstrating tangible and differentiated value of
the application of AI is critically important - now more than ever. The technology industry and Pharma R&D are at an inflection point - interests and outcomes can converge or diverge - depending on the value that AI can deliver.
12:50 Session Break
1:20 Dessert Refreshment Break in the Exhibit Hall with Poster Viewing
1:55 Chairperson’s Remarks
Bino John, PhD, Associate Director, AstraZeneca
2:00 FEATURED PRESENTATION: Application of Machine Learning and Artificial Intelligence as a Driver of Productivity in Drug Discovery & Development
Morten Sogaard, Vice President, Target Sciences & Technologies, External Sciences & Innovation, Worldwide R&D, Pfizer
This talk will provide an overview of the impact of AI on productivity on pharma with the focus on three areas – process engineering & automation, drug design and manufacturing, and target and biomarker discovery and validation, illustrated
by specific examples.
2:30 Intersection of AI Techniques and Rare Disease Diagnosis
Margaret Bray, PhD, Senior Data Scientist, Alexion
A look into the latest AI techniques applied to the field of rare disease diagnostics as well as a look at the limitation of current methodologies and areas for future growth.
3:00 Automated Compliance and Quality Checks
Etzard Stolte, PhD, Global Head Knowledge Management PTD, F. Hoffmann-La Roche
Machine learning technologies, like natural language processing (NLP), have reached the maturity for automated quality controls of operational information, e.g. as compliance and quality supervision tools. Over the last years Pharma Technical Development
at Roche has created a single front end for many business and validated systems that uses a mixture of curation and supervised learning tools to increase compliance and reduce operational costs. This talk will present our learnings, as well as the
limitations and opportunities we see for the future.
3:30 AI for Improving Drug Safety to Accelerate Drug Development
Bino John, PhD, Associate Director, AstraZeneca
Drug candidates that result from millions of dollars in investment frequently fail during clinical or preclinical testing phases due to safety concerns. Such safety related failures continue to pose a challenge to the industry. This talk will highlight
some of the efforts at AstraZeneca that seek to use AI/ML approaches to minimize such clinical/preclinical failures.
4:00 Conference Adjourns