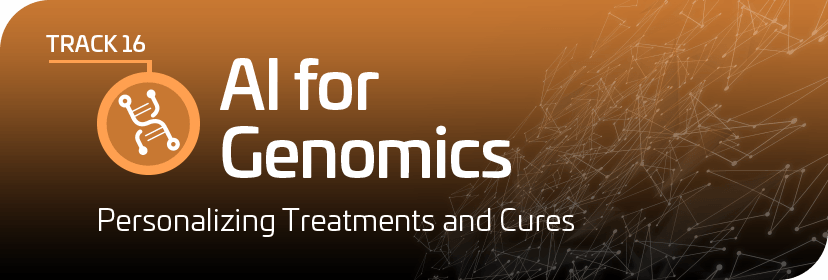
The role of computer science in modeling cells, analyzing and mapping data networks, and incorporating clinical and pathological data to determine how diseases arise from mutations is becoming more important in genomic medicine. We need to understand
where the disease starts and how artificial intelligence delivers genes and pathways for drug targets and diagnostics. The Inaugural AI for Genomics track explores case studies that apply deep learning, machine learning,
artificial intelligence, and predictive analysis methods to genomic medicine. We will discuss data curation techniques, text mining approaches, and statistical analytics that utilize deep machine learning to support AI efforts. This will help to integrate
omics approaches to discover disease or drug response pathways and identify personalized and focused treatments and cures.
Final Agenda
Tuesday, April 16
7:00 am Workshop Registration Open and Morning Coffee
8:00 – 11:30 Recommended Morning Pre-Conference Workshops*
W4. AI for Pharma
12:30 – 4:00 pm Recommended Afternoon Pre-Conference Workshops*
W12. Data Science Driving Better Informed Decisions
* Separate registration required.
2:00 – 6:30 Main Conference Registration Open
4:00 PLENARY KEYNOTE SESSION
amphitheater
5:00 – 7:00 Welcome Reception in the Exhibit Hall with Poster Viewing
Wednesday, April 17
7:30 am Registration Open and Morning Coffee
8:00 PLENARY KEYNOTE SESSION
Amphitheater
9:45 Coffee Break in the Exhibit Hall with Poster Viewing
ACCELERATING PRECISION MEDICINE WITH AI: CHALLENGES & OPPORTUNITIES
harborview 2
10:50 Chairperson’s Remarks
Richard Harrison, PhD, Products – Life Sciences, Senior Manager, Accenture
11:00 Next Generation Cognitive Supercomputing and Its Impact on Precision Medicine: Challenges, Trends and Opportunities
Edmon Begoli, PhD, Chief Data Architect, Oak Ridge National Laboratory
The impact of AI methods and big data technologies in pharma research and genomic medicine to advance precision medicine initiatives are taking on more importance. AI is becoming key player in the convergence of medical data and computer technologies.
This talk gives a big picture viewpoint on the evolvement of AI’s role, what the key drivers and challenges are, and trends to look for during the next 10-15 years.
11:30 From Hype to Reality: Data Science Enabling Personalized Medicine
Holger Fröhlich, PhD, Director and Head of Data Science Enablement, R&D Informatics, UCB BioSciences GmbH
Personalized, precision, P4, or stratified medicine is understood as a medical approach in which patients are stratified based on their disease subtype, risk, prognosis, or treatment response using specialized diagnostic tests. The key idea is to base
medical decisions on individual patient characteristics, including molecular and behavioral biomarkers, rather than on population averages. Personalized medicine is deeply connected to and dependent on data science, specifically machine learning (often
named Artificial Intelligence in the mainstream media). While during recent years there has been a lot of enthusiasm about the potential of ‘big data’ and machine learning-based solutions, there exist only few examples that impact current
clinical practice. The lack of impact on clinical practice can largely be attributed to insufficient performance of predictive models, difficulties to interpret complex model predictions, and lack of validation via prospective clinical trials that
demonstrate a clear benefit compared to the standard of care. In my talk, I will review the potential of state-of-the-art data science approaches for personalized medicine, discuss open challenges, and highlight directions that may help to overcome
them in the future.
12:00 pm Harness AI and Redefine Precision Medicine Infrastructure with Dell EMC
Sanjay Joshi, Industry CTO, Healthcare, Dell EMC, Global CTO Office, Dell EMC
Personalized, precision, P4, or stratified medicine is understood as a medical approach in which patients are stratified based on their disease subtype, risk, prognosis, or treatment response using specialized diagnostic tests. The key idea is to base
medical decisions on individual patient characteristics, including molecular and behavioral biomarkers, rather than on population averages. Personalized medicine is deeply connected to and d
12:30 Session Break
12:40 Luncheon Presentation: Productionising AI – Moving Beyond “Fire and Forget” to Predictable, Predictive Algorithms
Tim Miller, Vice President, Life Sciences Platform Solutions, Elsevier
Everybody is “doing AI” in Life Sciences right now, but how do we know we are doing it right? Are we picking the best models for our problem? Are we getting the quality of data we need? And, are we able to translate successful AI experiments
into productionised capabilities that integrate with our business goals?
1:10 NEW: Luncheon Presentation II: Enabling Perpetual Digital Transformation in Research & Development
Vikram Karakoti, Head, Life Sciences Business Unit, North America, Tata Consultancy Services
Life Sciences companies today are inverting the pyramid by embedding enabling technologies in business platforms. “Enabling Perpetual Digital Transformation in R&D” explores how these digital platforms are re-constructed, and coupled with
an “enterprise agile” R&D organization to yield exponential improvements in speed, capacity, cost, quality and improved outcomes in product research and clinical development; with real-life examples of just how the new paradigm is
being embraced by leading Life Sciences companies.
1:40 Session Break
1:50 Chairperson’s Remarks
Bino John, PhD, Associate Director, AstraZeneca
1:55 Reduction of scRNA-seq by Deep Learning
Shanrong Zhao, PhD, Director, Computational Biology and Bioinformatics, Pfizer
2:25 CRISPR On- and Off-target Prediction and Evaluation
Andrew Kernytsky, PhD, Director, Head of Genomics and Computational Biology, CRISPR Therapeutics
This talk will look at evaluating off-target (mostly) prediction methods with some real lab data and assess what existing ML can do vs. what's left to improve upon and how (layering epigenetics et al.).
2:55 Prototype ML Software for Analysis of RNAseq, and Development of RNAseq for ML-ready Applications Developed in NIH-Hackathons!
Ben Busby, PhD, Scientific Lead, NCBI Hackathons Group, National Center for Biotechnology Information (NCBI)
3:25 Refreshment Break in the Exhibit Hall with Poster Viewing, Meet the Experts: Bio-IT World Editorial Team, and Book Signing with Joseph Kvedar, MD, Author, The Internet of Healthy Things℠ (Book will be available
for purchase onsite)
4:00 Transformative Gene Therapies for Severe Genetic Diseases and T Cell-Based Immunotherapies
Hans Bitter, PhD, Vice President, Data Sciences, bluebird bio
4:30 Deep Learning for Precision Medicine: Emerging Technology, Trends, and Potential
Gaurav Kaushik, Co-Founder, Cascade Bio, Inc.
Deep learning methods have transformed modern life; they shape how we engage online, the products we purchase, the news and information we gather, and more. The transformation of precision medicine is not far behind. AI-embedded health and technology
products will reshape how we think about data analysis, evidence generation, and information synthesis. This talk will provide an overview of emerging deep learning methods, with deep dives into specific architectures and practical applications,
trends to watch in the near future, and opportunities for individuals to leverage deep learning across various aspects of precision medicine, such as variant calling, clinical trial recruitment, information synthesis and recall, and more. This
talk is for all audiences -- newcomers to deep learning who wish to know more are encouraged to attend.
5:00 AI for Genomics is the Answer, but What is the Question?
Jonathan Usuka, PhD, MBA, Senior Expert, McKinsey & Company
State-of-the-art bioinformatics has caught up with the challenges of yesterday’s Pharmaceutical R&D needs, which involved automated workflows, gene annotations, and pathway-based target identification. But Today’s Pharmaceutical R&D
is undergoing a fundamental transformation. New modalities based on Immuno-Oncology and CRIPSR, the ascendance of personalized therapies, and the incorporation of real-world data are overwhelming solutions designed for yesterday’s genomics
analysis. Where do we go from here?
5:30 Best of Show Awards Reception in the Exhibit Hall with Poster Viewing
Thursday, April 18
7:30 am Registration Open and Morning Coffee
8:00 PLENARY KEYNOTE SESSION & AWARDS PROGRAM
Amphitheater
9:45 Coffee Break in the Exhibit Hall and Poster Competition Winners Announced
10:30 Chairperson’s Remarks
Chris Anderson, Editor in Chief, Clinical OMICs
10:40 Genomic Prediction of Complex Traits and Disease Risks via Machine Learning and AI
Stephen Hsu, PhD, Senior Vice President for Research and Innovation and Professor of Theoretical Physics, Michigan State University
After a brief review (suitable for non-specialists) of computational genomics and complex traits, I describe recent progress in this area. Using methods from Machine Learning / AI, and data sets such as the UK BioBank 500k SNP genotypes, we construct
genomic predictors for several complex traits. Our height predictor captures nearly all of the predicted SNP heritability for this trait -- actual heights of most individuals in validation tests are within a few cm of predicted heights. I also
discuss application of these methods to cognitive ability and polygenic disease risk. We can now identify risk outliers for conditions such as heart disease, diabetes, breast cancer, hypothyroidism, etc. using inexpensive genotyping. Finally,
I discuss how these advances will affect human reproduction (embryo selection for in vitro Fertilization (IVF); gene editing) in the coming decade.
11:10 Precision Medicine in the Age of Data: The Evolution of Oncology, Genomics, and Real-World Evidence
Gaurav Singal, MD, Chief Data Officer, Foundation Medicine, Inc.
11:40 Tackling the Clinical Data Challenges When Analyzing a Million Genomes
Kees van Bochove, CEO, The Hyve
Population genetics and genomics is an emerging topic for the application of machine learning methods in healthcare and biomedical sciences. Currently, several large genomics initiatives, such as Genomics England, UK Biobank, the All of Us Project,
and Europe's 1 Million Genomes Initiative are all in the process of making both clinical and genomics data available from large numbers of patients to benefit biomedical research. However, a key challenge in these initiatives is the standardization
of the clinical and outcomes data in such a way that machine learning methods can be effectively trained to discover useful medical and scientific insights. In this talk, we will look at what data is available at scale, and review some of examples
of the application of common data and evidence models such as OMOP, FHIR, GA4GH, RADAR-BASE and OpenTargets in order to achieve this, based on projects which The Hyve has executed with some of these initiatives to harmonize their clinical, genomics,
imaging and wearables data and make it FAIR.
12:10 pm Session Break
12:20 Enjoy Lunch on Your Own (Lunch Available for Purchase in Exhibit Hall)
1:20 Dessert Refreshment Break in the Exhibit Hall with Poster Viewing
1:55 Chairperson’s Remarks
Yuval Itan, PhD, Assistant Professor, Department of Genetics and Genomic Sciences; Member, Charles Bronfman Institute for Personalized Medicine, Icahn School of Medicine at Mount Sinai
2:00 Pinpointing Transcript-Damaging Disease-Causing Variants as a Major Step towards RNA Therapeutics
Sahar Gelfman, PhD, Associate Research Scientist, Columbia University Medical Center
The difficulty in capturing pathogenic variants that indirectly damage mRNA formation results in overlooking synonymous and intronic variants when searching for disease risk in sequenced genomes. The Transcript-inferred Pathogenicity (TraP) model
was developed to identify sequence context changes that affect splicing decisions and the formation of the final transcript. A random forest model is trained on previously described pathogenic and benign synonymous mutations and identifies damaging
variants with over 97% specificity and with a sensitivity three-four times higher than other available scores. Importantly, the specific mode of action of TraP damaging variants can be rescued using carefully designed small molecules, thus identifying
these variants is a big step towards personalized treatments for mutation carriers. Since its publication in 2017, TraP has become a major resource for genetic diagnostics that is helping to change the common conception that pathogenic genetic
variation is caused solely by coding mutations. TraP has been incorporated in diagnostic pipelines in tens of research institutes worldwide, among which are the NIH, Nationwide Children’s Hospital, SickKids foundation, Massachusetts General
Hospital and others. TraP is also available as a website for single queries (www.trap-score.org) that is used systematically by over 1,500 users from clinics and genetic institutes in over 40 countries
worldwide, providing successful diagnosis of genetic disorders and affecting treatment decisions.
2:30 AI Assisted Rapid Clinical Whole Genome Sequencing for Critical Care
Ray Veeraraghavan, PhD, Director of IT & Informatics, Rady Children's Institute for Genomic Medicine
3:00 Deciphering the Complex Heterogeneity of Cancer
Patrice M. Milos, PhD, Co-Founder/President and CEO, Medley Genomics, Inc.
In 2017, 1.7 million people in the US were diagnosed with cancer, and even though cancer survival rates have increased, it still accounts for 1 in 4 deaths annually. Cancer, a heterogeneous disease, has significant tumor cell variability within individual
patients, as well as across categories of patients, creating complex barriers to effective and lasting cures for patients. Understanding this heterogeneity will be required to individualize care for patients. Medley Genomics provides a software
platform that uses patent-pending algorithms and advanced data analytics to describe a patient's diverse tumor cell mixture. This enables creation of unique molecular diagnostic fingerprints for improving patient diagnosis, monitoring and treatment
of cancer, and helps to improve novel oncology therapies and therapeutic combinations including individual cancer vaccine development.
3:30 Estimating Genotypic Heterogeneity Underlying Human Disease
Yuval Itan, PhD, Assistant Professor, Department of Genetics and Genomic Sciences; Member, Charles Bronfman Institute for Personalized Medicine, Icahn School of Medicine at Mount Sinai
Whole exome and whole genome sequencing provide hundreds of thousands of genetic variants per patient, of them only very few are pathogenic. Current computational methods are inefficient in differentiating pathogenic mutations from neutral genetic
variants that are predicted to be damaging, and cannot predict the functional outcome of mutations. We will present: (1) a deep learning approach to efficiently detect pathogenic mutations by utilizing extensive annotations and patients’
phenotypic data; (2) a machine learning method combined with natural language processing to estimate whether a mutation results in gain- or loss-of-function; and (3) a cases-controls gene burden study to detect genes and pathways enriched with
rare and high impact disease-causing mutations in exomes of over 2,000 Ashkenazi Jewish patients suffering from inflammatory bowel disorder. Finally, we will present new tools to visualize and extract useful information of human, mutations, and
DNA/protein sequences for better utilization of next generation sequencing data and understanding of human disease genomics.
4:00 Conference Adjourns